New Delhi, Jan 5 (IANS): The Google DeepMind robotics team has introduced new AI-based systems based on large language models (LLMs) to help develop better multi-tasking robots for our daily use.
The tech giant unveiled AutoRT, SARA-RT and RT-Trajectory systems to improve real-world robot data collection, speed, and generalisation.
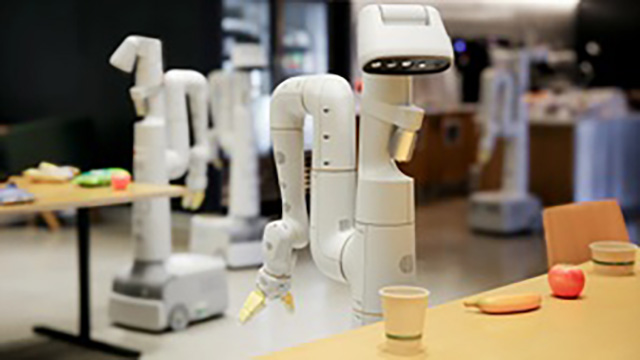
“We’re announcing a suite of advances in robotics research that bring us a step closer to this future. AutoRT, SARA-RT, and RT-Trajectory build on our historic Robotics Transformers work to help robots make decisions faster, and better understand and navigate their environments,” the Google DeepMind team said in a statement.
AutoRT harnesses the potential of large foundation models which is critical to creating robots that can understand practical human goals.
By collecting more experiential training data AutoRT can help scale robotic learning to better train robots for the real world, said the team, said Google.
AutoRT combines large foundation models such as a LLM or a Visual Language Model (VLM), and a robot control model (RT-1 or RT-2) to create a system that can deploy robots to gather training data in novel environments.
“In extensive real-world evaluations over seven months, the system safely orchestrated as many as 20 robots simultaneously, and up to 52 unique robots in total, in a variety of office buildings, gathering a diverse dataset comprising 77,000 robotic trials across 6,650 unique tasks,” the team informed.
The Self-Adaptive Robust Attention for Robotics Transformers (SARA-RT) system converts Robotics Transformer (RT) models into more efficient versions.
“The best SARA-RT-2 models were 10.6 per cent more accurate and 14 per cent faster than RT-2 models after being provided with a short history of images. We believe this is the first scalable attention mechanism to provide computational improvements with no quality loss,” said the DeepMind team.
When the team applied SARA-RT to a state-of-the-art RT-2 model with billions of parameters, it resulted in faster decision-making and better performance on a wide range of robotic tasks.
Another model called RT-Trajectory hich automatically adds visual outlines that describe robot motions in training videos.
RT-Trajectory takes each video in a training dataset and overlays it with a 2D trajectory sketch of the robot arm’s gripper as it performs the task.
“These trajectories, in the form of RGB images, provide low-level, practical visual hints to the model as it learns its robot-control policies,” said Google.
When tested on 41 tasks unseen in the training data, an arm controlled by RT-Trajectory more than doubled the performance of existing state-of-the-art RT models: it achieved a task success rate of 63 per cent compared with 29 per cent for RT-2.
“RT-Trajectory can also create trajectories by watching human demonstrations of desired tasks, and even accept hand-drawn sketches. And it can be readily adapted to different robot platforms,” according to the team.